Hello everyone, I read a survey paper on number representation in NLP, encounter a GPT-3 replica model in huggingface model hub, and project Starline. Here few short notes on those.
Representing Numbers in NLP: a Survey and a Vision [paper]
Numbers are important and integral part of text, and yet numbers rarely get special consideration when processing text. Many NLP systems are commonly treated numbers as words, and subword tokenization such as BPE breaks numbers into arbitrary tokens, for example 1234 might be splitted into 1-234 or 12-34 or 123-4.
This paper surveys recent numeracy work in NLP and categorized them into seven numeracy tasks:
- Simple arithmetic: arithmetic operation such as addition, subtraction over number alone.
- Numeration: decoding a string form to its numeric value.
- Magnitude comparison: ability to perform comparison on two or more numbers.
- Arithmetic word problems: ability to perform simple arithmetic from a composition.
- Exact facts: understanding numbers in commonsense knowledge.
- Measurement estimation: approximately guess measure of objects along certain dimensions.
- Numerical language modeling: making numeric predictions in completing text.
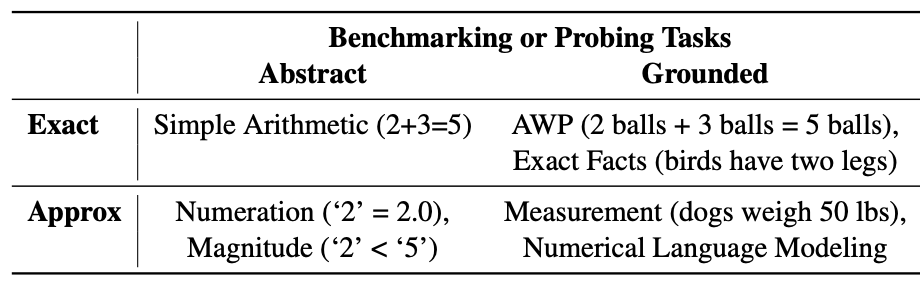
The numeracy tasks are categorized following two dimensions:
- Granularity. Whether the encoding of the number is exact or approximate.
- Units. Whether the numbers are abstract or grounded.
The author groups number representation into string-based and real-based representation. String-based representation treat numbers as strings, with several tweaks. Real-based representation performs computation using the numerical value of the number. Detail summary on each representation can be read in the paper.
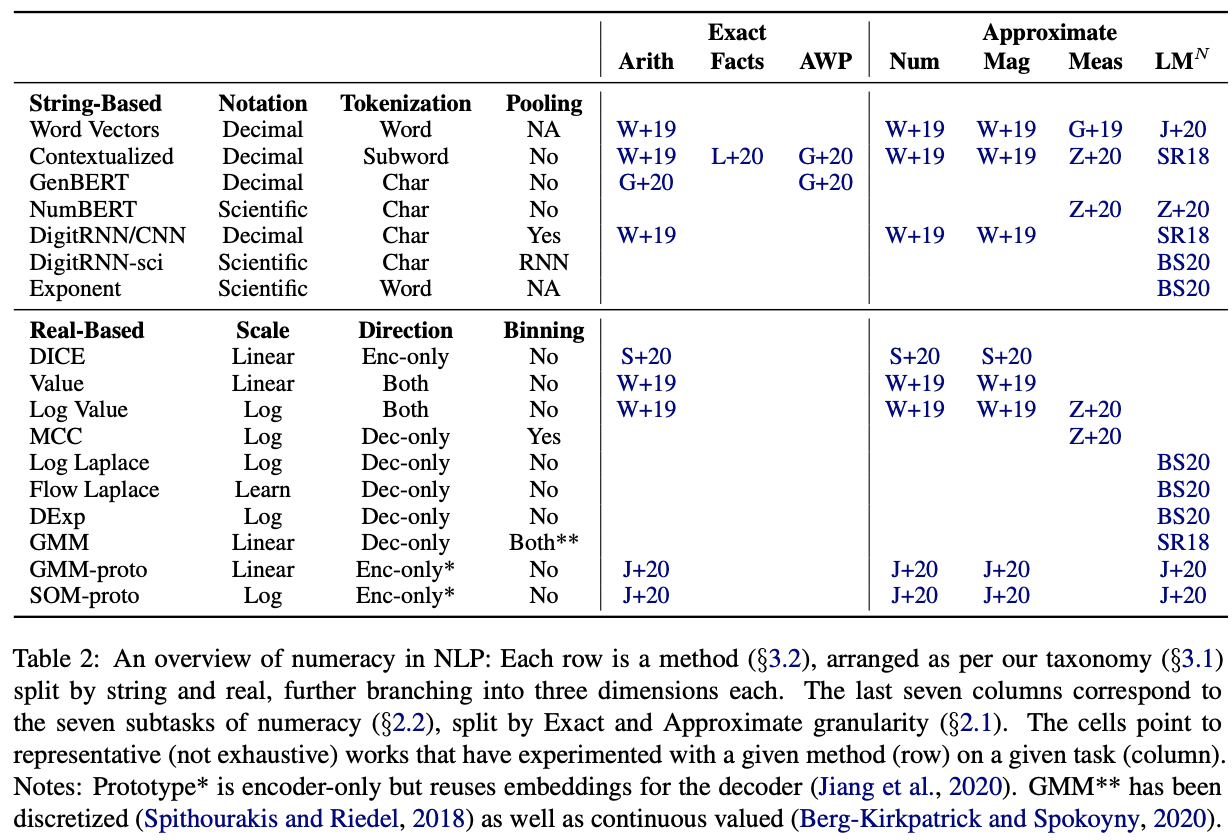
The authors present few practical takeaways to guide the design of number representation:
- For string-based representation:
- Scientific notation is superior to decimal notation
- Character level tokenization outperforms subword level tokenization.
- For real-based representation:
- Log scale is preferred over linear scale as inspired by cognitive science literature (but it lacks of rigorous study)
- Binning (dense cross entropy loss) works better than continuous value prediction (MAE loss)
They also call for unified and more holistic solution to numeracy. This involves a benchmark covering different numeracy subtasks to incentive research progress in numeracy.
GPT-Neo: GPT-3 Replica by EleutherAI [model][article]
OpenAI GPT-3 has been powering many applications in various domains from creativity to productivity. Through OpenAI API, GPT-3 generates about 4.5 billions words per day. However, access to the OpenAI API is not free and still very limited to few companies and developers. And, training GPT-3 from scratch demands a lot of computing power that most people cannot afford.
EleutherAI replicates GPT-3 architecture and trains the model on the Pile dataset. The Pile dataset is 825GB open source data for language modeling, combining texts from 22 sources such as English Wikipedia, OpenWebText2, PubMed Central, ArXiv, Github, Stack Exchange, Ubuntu IRC, and the US Patent and Trademark Office.
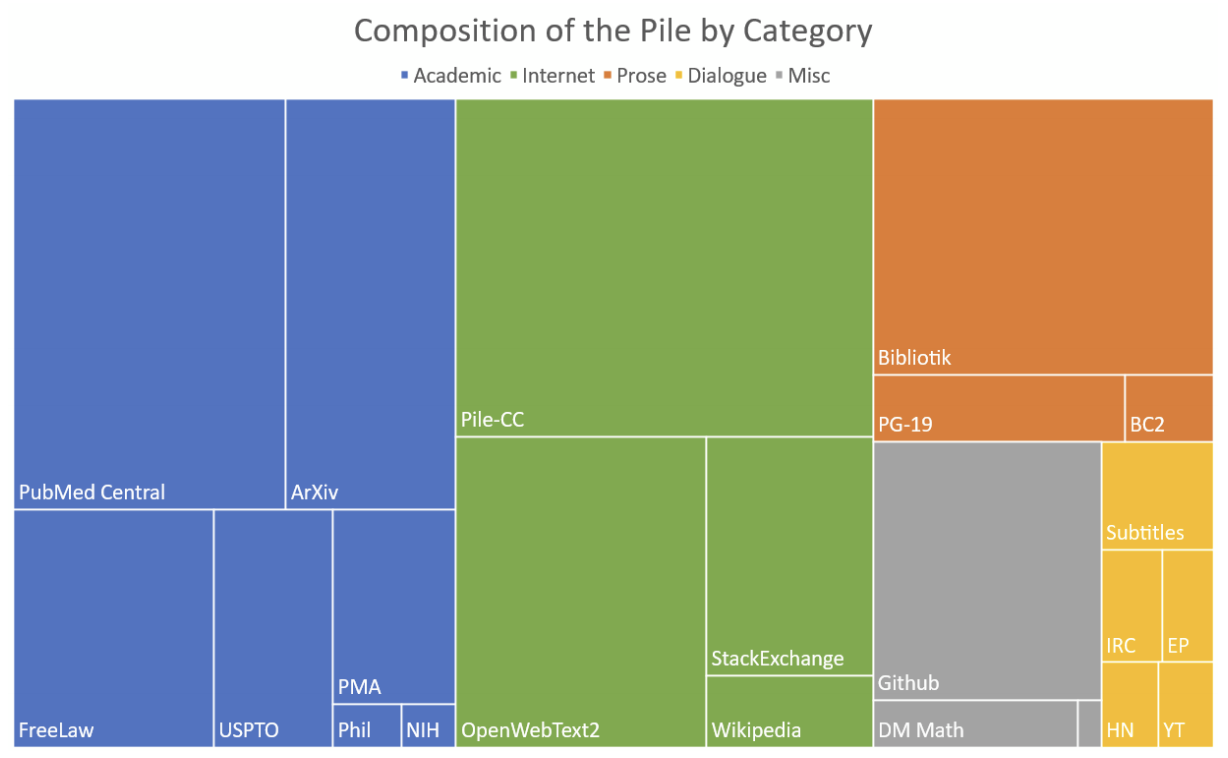
The trained model is called GPT-Neo. The model has 2.7B parameters, and it is comparable to the smallest GPT-3 model. This model is a great free alternative to GPT-3, and it is available in the huggingface model hub.
Project Starline [blog]
Imagine having a long-distance conversation with your loved one, but you can see the person in real-life size, and three dimensions though a sort of magic windows. Google research applies technologies in computer vision, machine learning, spatial audio and real time compression, as well as light field display system to create a magic window that gives a sense of volume and depth without the needs for additional headsets or glasses. The result is a feeling of a person right in front of you, just like he/she is right there.
That’s all for this week. Stay safe and see you next week!