For this week in machine learning, I am sharing two interesting tutorials from VLDB and KDD conferences this week.
Managing ML Pipelines: Feature Stores and the Coming Wave of Embedding Ecosystems [slides][paper]
Machine learning pipeline is an iterative process involving data curation, feature engineering, training and deploying models, as well as monitoring and maintenance of the deployed models. In large system with many downstream tasks, a feature store is important to standardize and manage feature generation and workflows in using the features. With the advent of self-supervised pre-trained embedding models as features, feature store faces new challenges to manage embeddings.
This VLDB 2021 tutorial gives an overview of the machine learning pipeline and feature store. Then, it introduces embeddings and the challenges faced by feature store in dealing with embeddings. Finally, it introduces recent solutions to some of the challenges and discussion on the future direction.
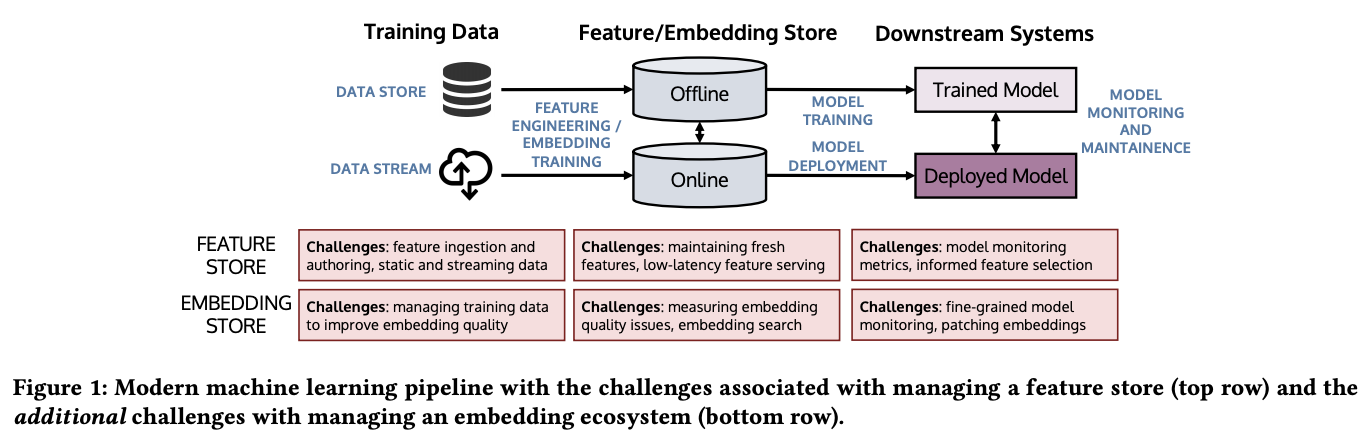
All You Need to Know to Build a Product Knowledge Graph [website]
This tutorial by Amazonian at KDD 2021 presents best practices in building scalable product knowledge graph. Building product knowledge graph is more challenging than generic knowledge graph due to the sparsity of the data, the complexity of the product domains, evolving taxonomies, and noise in the data.
The tutorial covers the solutions to answer the challenges in building product knowledge graph including knowledge extraction, knowledge cleaning, and ontology construction. Finally, it concludes with some practical tips and future directions.
That’s all for this week. Stay safe, and see you next week.